Demo code for EEG/MEG connectivity analysis
This demo script gives an example on:
- calculating and visualizing the imaginary part of the cross-spectrum (ImCs) and coherency (ImCoh) for EEG (analog for MEG) data on sensor level.
- the Global Interaction Measure (GIM) to find frequency peaks of ongoing synchronization effects within the data
- the "corrected" imaginary part of coherency (cImCoh)
- SC-MUSIC to localize synchronized sources at a particular frequency
- the Multivariate Interaction Measure (MIM) and the Maximized Imaginary Coherence (MIC) in different application scenarios:
- to fix the dipole direction of a prior inverse filtering step (here done by LCMV beamforming, but could be something like eLoreta as well) with respect to the connectivity to a reference source
- to calculate the connectivity between brain regions defined by an anatomical atlas (here AAL)
- to calculate the connectivity between groups of sensors and visualize the spatial patterns
(Please note that MIM can be seen as the total connectivity between two spaces whereas MIC is the maximized connectivity - both in terms of the imaginary part of coherency, and, hence, robust to volume conduction artifacts)
6. Wedge MUSIC to identify subsystems of interacting sources (which of the SC MUSIC source is interacting with which other source?). Wedge MUSIC (and SC-MUSIC) are particularly suited to investigate class differences. Here, Wedge MUSIC is exemplarily shown in three variants: scan, scalar, complete.
The corresponding Matlab® m-files and the simulated demo data can be found under http://www.aewald.net/code
History of this demo script (current version 1.0):
- 01.08.2015: initial version by Arne Ewald (mail@aewald.net)
Contents
- Preparation
- 1. Estimating the cross-spectrum and coherency
- 2. Imaginary part of coherency (ImCoh) / Global Interaction Measure (GIM) / 'corrected' ImCoh (cImCoh)
- 3. Self-Consistent MUSIC (SC-MUSIC)
- 4. LCMV beamforming + MIM to fix the dipole orientation
- 5. MIM between AAL regions (with LCMV beamformer)
- 6. MIM on sensor level
- 7. Wedge MUSIC
Preparation
Add the path of the files and the data to your Matlab® search path (This you have to adapt to your individual folder settings):
restoredefaultpath
addpath(genpath('C:\Dokumente\demo1\'))
Load the simulated data for 56 EEG channels with two phase (~=0) synchronized brain sources. The data have been modeled in source space with a 4-th order auto-regressive (AR) model with the following non-zero coefficients:
A1(1,1)=.45; A4(1,1)=-.65; A1(2,2)=.45; A2(2,2)=-.9; A2(2,1)=1;
These coefficients lead to a coherent signal (with a time delay) at 10Hz as often observable in EEG or MEG data (alpha band). However, this way of modeling the data does not lead to a 1/f powerspectrum (which is also not necessary for the demonstration purposes here). Additionally, random Gaussian noise for each source voxel has been added to the modeled data with twice the power of the noise-free signal. Finally, these source time series have been projected to sensor level.
load data56channels.mat
[nt, nch]=size(data);
Format: samples x channels Name Size Bytes Class data 50000x56 22400000 double
Load information for later source analysis (sa):
load sademo1.mat;
Format: sa = mri: [1x1 struct] -> MRI structure for plotting V_coarse: [56x2113x3 double] -> lead fields (channels x voxels x 'xyz'-direction) grid_coarse: [2113x3 double] -> grid coordinates locs_2D: [56x5 double] -> 2D coordinates of electrodes clab_electrodes: {1x56 cell} -> electrode names (10-20 system)
Showing the position of the initially modeled dipoles:
true_dipoles = [5 0 4 1 1 1; ... -5 0 4 -1 1 1]; source_pars = struct('orientation', 'coronal', ... 'ncol', [1 1], ... 'mricenter', [-2 0 11], ... 'dslice_shown', 5, ... 'mydipmarkersize', 1.5, ... 'mydiplinewidth', 2.5, ... 'trcut', 0.25, 'colorbars', 0); figure; showmri_transp_v31(sa.mri, source_pars, true_dipoles); % some figure scaling: set(gcf,'PaperUnits','centimeters') foffset=100; xSize = 6; ySize = 6; xLeft = (21-xSize)/2; yTop = (30-ySize)/2; set(gcf,'PaperPosition',[xLeft yTop xSize ySize]) set(gcf,'Position',[foffset foffset xSize*50 ySize*50])
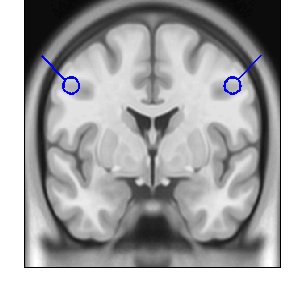
1. Estimating the cross-spectrum and coherency
Parameter for spectral estimation
The sampling frequency of the modeled data is assumed to be 100 Hz:
fs=100;
To calculate the cross-spectrum or coherency, the data are averaged over trials (or epochs). The variable epleng determines the length of one epoch in the data (the data are assumed to be concatenated trials. However, one can perform the same analysis for resting state data) :
epleng=500;
As the number of sampling points is nt=50000, an epoch length of epleng=500 results in 100 epochs and each epoch contains 5 seconds of data. For sufficiently long epochs, each epoch can again be subdivided into segments. This might be meaningful e.g. to calculate time resolved event-related power. Here, we use segments to average over them within one epoch. This leads to a larger number of averages in total and makes the frequency estimates more reliable.
Therefore, one can define a segment length
segleng=100;
which also determines the frequency resolution. In this example, where segleng = fs the frequency resolution is 1Hz.
In each segment(!) a Hanning windowed FFT will be computed and averaged over segments within each epoch. Furthermore, it will be averaged over all epochs. The variable 'segleng' determines the resulting frequency resolution. Examples for fs=100Hz:
- segleng=100 leads to a frequency resolution of 1Hz. The outcome of 'data2cs_event.m' would be 51 frequency bins (due to Nyquist). 50Hz corresponds to frequency bin 51 and 13Hz to Frequency bin 14.
- segleng=50 data points leads to a frequency resolution of 2Hz. The outcome of 'data2cs_event.m' would be 26 frequency bins (due to Nyquist). 50Hz corresponds to frequency bin 26.
- segleng=200 data points leads to a frequency resolution of 0.5Hz. The outcome of 'data2cs_event.m' would be 101 frequency bins (due to Nyquist). 50Hz corresponds to frequency bin 101.
With the variable segshift, one can define a sliding window approach. Here, we do not use a sliding window approach and set:
segshift=segleng;
An alternative would be segshift=segleng/2 which would double the number of averages. The spectrum will always be calculated up to the Nyquist frequency fs/2 unless maxfreqbin is set to something different (smaller than fs/2):
maxfreqbin=(segleng/2)+1;
One can also define additional parameters:
para=[]; para.segave = 1; % average over segments ? para.subave = 0; % subtract average ?
The frequency resolution can be calculated as
df=fs/segleng; xf=(0:maxfreqbin-1)*df;
Calculating the cross-spectrum and coherency
[cs, coh, nave]=data2cs_event(data, segleng, segshift, epleng, maxfreqbin, para);
cs: complex valued cross-spectrum (chans x chans x frequency bins) coh: complex valued coherency (chans x chans x frequency bins) nave: number of averages (1 x 1)
Plotting
One can plot coherence, i.e. the absolute value of coherency, over frequency for all channel pairs:
figure; plot(xf(1:maxfreqbin), reshape(abs(permute(coh(:,:,1:maxfreqbin),[3 2 1])),maxfreqbin, nch*nch)); % setting the x axis label such that it corresponds to Hz label_step=5; set(gca,'XTick',1:label_step:maxfreqbin); set(gca,'XTickLabel',(0: label_step :maxfreqbin)); xlabel('Frequency [Hz]'); ylabel('Coherence for all channel pairs'); % some figure scaling: set(gcf,'PaperUnits','centimeters') foffset=100; xSize = 12; ySize = 8; xLeft = (21-xSize)/2; yTop = (30-ySize)/2; set(gcf,'PaperPosition',[xLeft yTop xSize ySize]) set(gcf,'Position',[foffset foffset xSize*50 ySize*50])
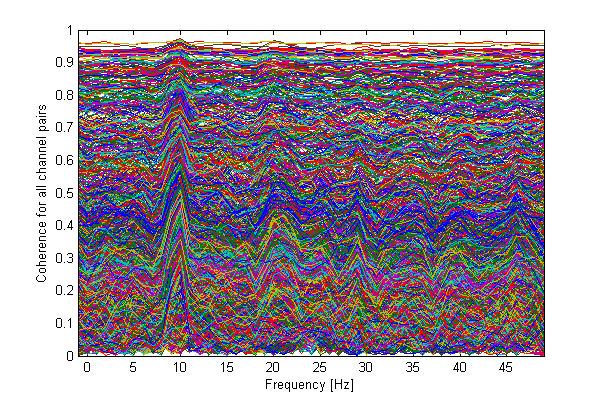
Visualization of coherence as a 'head-in-head-plot' at 11 Hz. For each sensor the relations (here: coherence to each other sensor is color-coded
figure; showcs(abs(coh(:,:,12)), sa.locs_2D);
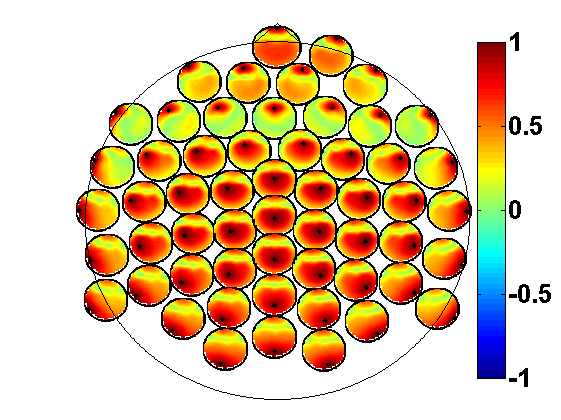
2. Imaginary part of coherency (ImCoh) / Global Interaction Measure (GIM) / 'corrected' ImCoh (cImCoh)
The coherence based head-in-head-plot (see above) mainly shows artifacts of volume conduction. Basically, each electrode signal is highly coherent to signals form neighboring electrodes.
As a remedy, one can look at the imaginary part of coherency (ImCoh) for all channel pairs as the ImCoh neglects instantaneous synchronization effects.
see Nolte, et. al. 2004: Nolte, G., Bai, O., Wheaton, L., Mari, Z., Vorbach, S., Hallett, M., 2004. Identifying true brain interaction from EEG data using the imaginary part of coherency. Clinical Neurophysiology 115 (10), 2292-2307.
Furthermore, one can study the Global Interaction Measure (GIM)
see Ewald, et. al. 2012: Ewald, A., Marzetti, L., Zappasodi, F., Meinecke, F. C., Nolte, G., 2012. Estimating true brain connectivity from EEG/MEG data invariant to linear and static transformations in sensor space. NeuroImage 60, 476 488. URL http://www.sciencedirect.com/science/article/pii/S1053811911013668
The GIM can be seen as the total interaction robust to volume conduction. As shown in Ewald, et. al. 2012, it is derived by maximizing the ImCoh which leads to an increase in signal-to-noise ratio. Hence, with the GIM, synchronization effects might become more prominent compared to pair-wise ImCoh. The GIM is calculated by
pp=15; % a parameter controlling for overfitting [gim, bias]= proc_gim(cs, pp, nave); [~, idxg]=max(gim-bias); % frequency bin with maximum GIM for further analysis
The ImCoh for all channel pairs and the GIM can be visualized with
fig=figure; plot(xf(1:maxfreqbin), reshape(imag(permute(coh(:,:,1:maxfreqbin),[3 2 1])),maxfreqbin, nch*nch)); hold on h1=plot(xf, gim-bias, '--k', 'LineWidth', 5); hold off % setting the x axis label such that it corresponds to Hz label_step=5; set(gca,'XTick',1:label_step:maxfreqbin); set(gca,'XTickLabel',(0: label_step :maxfreqbin)); xlabel('Frequency (Hz)'); ylabel('Imaginary part of Coherency for all channel pairs'); legend(h1,'GIM'); legend('boxoff')
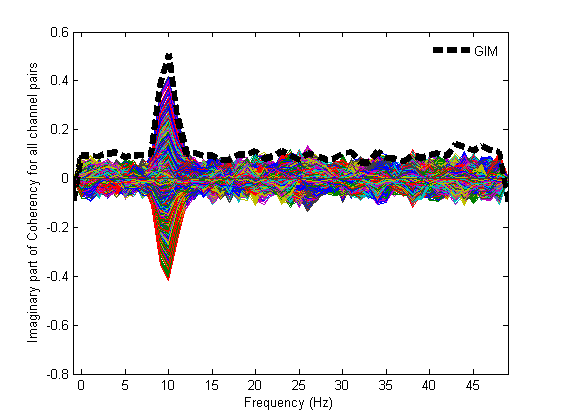
Visualization of the ImCoh as a 'head-in-head-plot' at the peak frequency. For each sensor the relations to each other sensor is color-coded
figure; showcs(imag(coh(:,:,idxg)), sa.locs_2D);
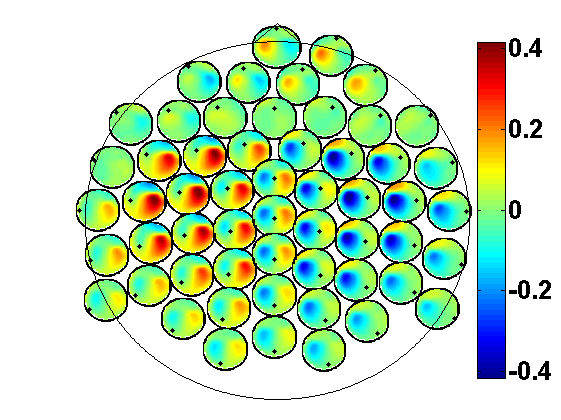
please note: in this example you can unravel the structure of the two underlying dipoles in this head in head plot. In practice that might not be so clear.
As has been shown in Ewald et. al. 2012, the regular ImCoh suppresses interactions in the vicinity of the reference. This effect is removed by using the 'corrected' ImCoh which can be calculated and visualized as follows:
cicoh=cs2cicoh(cs(:,:,idxg)); figure; showcs(cicoh, sa.locs_2D);
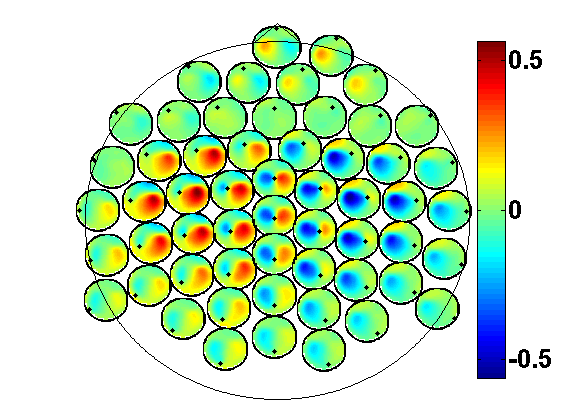
3. Self-Consistent MUSIC (SC-MUSIC)
For the loclization of synchronized sources SC-MUSIC is used here. see Shahbazi F., Ewald A., Nolte G., 2015: Self-Consistent MUSIC: An approach to the localization of true brain interactions from EEG/MEG data NeuroImage, in press http://www.sciencedirect.com/science/article/pii/S105381191500155X
[nch,nv,ndim]=size(sa.V_coarse); ns=2; %estimated number of sources % construct data subspace from the imaginary part of the cross-spectrum [u,s,v]=svd(imag(cs(:,:,idxg))); u0=u(:,1:ns); [s_all,vmax_all,imax_all,dips_mom_all,dips_loc_all]=sc_music(u0,sa.V_coarse,ns,sa.grid_coarse);
results in
s_all: source distributions (voxels x sources) vmax_all: source topographies (channels x sources) imax_all: index of source in grid (sources x 1) dips_mom_all: dipole moments (sources x 3) dips_loc_all: dipole locations (sources x 3)
To judge the performance of SC-MUSIC in this simulation, one can compare the locations of the originally modeled dipoles
disp(true_dipoles(:,1:3));
5 0 4 -5 0 4
and the estimated locactions
disp(dips_loc_all);
-5.0000 0.0000 4.0000 5.0000 0.0000 4.0000
4. LCMV beamforming + MIM to fix the dipole orientation
First, one has to construct the beamforming filter:
[A, A1, po]=mkfilt_lcmv(sa.V_coarse,cs(:,:,idxg));
resulting in
A : 3-dimensional filter (channels x voxels x 3) A1 : 1-dimensional filter along direction with strongest power (channels x voxels) po : Mx1 vector for M voxels, po(i) is the power at the i.th voxel along stronges direction (voxels x 1)
However, to look at a power for a single data class, one has to normalize, e.g. by:
L=reshape(sa.V_coarse,nch,[]);
C0=L*L';
[~, ~, pox]=mkfilt_lcmv(sa.V_coarse,C0);
%[~, ~, pox]=mkfilt_lcmv(sa.V_coarse,eye(nchan)); % different normalization
Now, one can plot power in source space:
source_pars = struct('orientation', 'all', ... 'nsubplotrows', 1, ... 'colorbars', 0); % [m, idxm]=max(po./pox); % source_pars.mricenter=ceil(sa.grid_coarse(idxm,:)); source_pars.mricenter=true_dipoles(1,1:3); figure; showmri_transp_v31(sa.mri,source_pars,[sa.grid_coarse,po./pox]); % some figure scaling: set(gcf,'PaperUnits','centimeters') foffset=100; xSize = 10; ySize = 3; xLeft = (21-xSize)/2; yTop = (30-ySize)/2; set(gcf,'PaperPosition',[xLeft yTop xSize ySize]) set(gcf,'Position',[foffset foffset xSize*50 ySize*50])
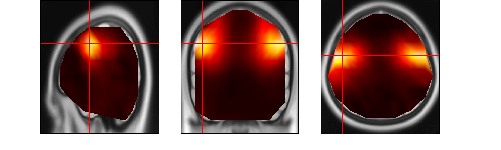
However, choosing the dipole direction with strongest power might not be the most reasonable approach, especially for the study of functional connectivity. A different approach would be to choose the dipole direction which provides strongest connectivity (in terms of ImCoh) with respect to a reference source. Here, A(:,imax_all,:) is the filter for the two sources obtained from SC-MUSIC. These are used as a reference sources (or seeds) here. Of course, a reference source can be e.g. any region of interest (ROI). To calculate the connectivity of the seed to all other voxels one can use
[mim_all,mic_all]=cs2nodes_mim(cs(:,:,idxg),A,A(:,imax_all,:));
resulting in
mim_all - MIM for all voxel pairs (voxels x references) mic_all - maximized ImCoh (MIC) (voxels x references)
To visualize the two interacting sources, one can use the following code and observe that the sources match the locations of the modeled dipoles.
source_pars = struct('orientation', 'all', ... 'nsubplotrows', 2, ... 'mymarkersize', 10, ... 'colorbars', 1); source_pars.mricenter=true_dipoles(1,1:3); figure; showmri_transp_v31(sa.mri,source_pars,[sa.grid_coarse,mim_all(:,1)], true_dipoles); source_pars.mricenter=true_dipoles(2,1:3); source_pars.subplotstart=4; showmri_transp_v31(sa.mri,source_pars,[sa.grid_coarse,mim_all(:,2)], true_dipoles);
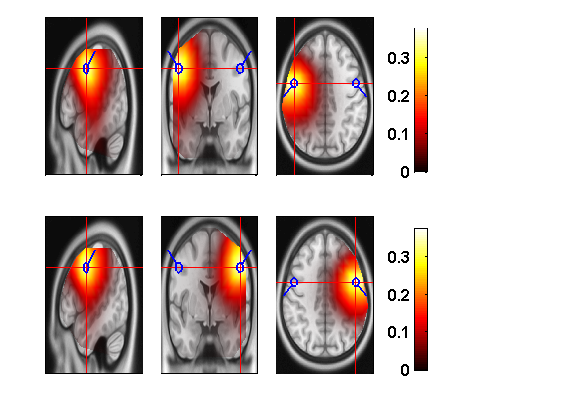
5. MIM between AAL regions (with LCMV beamformer)
A further approach to use the Multivariate Interaction Measure (MIM) is to estimate the connectivity between pre-defined anatomical regions containing multiple voxels. This can be done as follows:
% load AAL Atas load('aalmask_grid_coarse.mat'); load('aal_tissuelabel.mat'); nregs=90; [~, ngrid, ~]=size(sa.V_coarse); regs=unique(aalgrid.mask); regs(regs==0)=[]; mimaal=zeros(nregs,nregs); % region1 for rr1=1:nregs % region 2 for rr2=1:nregs % voxel indices of region 1 vir1=find(aalgrid.mask==rr1); % voxel indices of region 2 vir2=find(aalgrid.mask==rr2); if ((~isempty(vir1))&&(~isempty(vir2))) fr1=A(:,vir1,:); fr2=A(:,vir2,:); % because the coarse grid is used, it might happen that only a single % voxel is included in one region. Then, using the overfitting % parameter p=2 is invalid and the warning "Overfitting parameter not valid" % will be displayed. Therefore, the warnings are shortly turned % on and off in this demo now. warning('off','all'); [mimaal(rr1,rr2)]=cs2reg_mim(cs(:,:,idxg), fr1, fr2, 2); warning('on','all') end % if end % for reg 1 end % for reg 2
plotting the resulting connectivity matrix:
figure; imagesc(tril(mimaal)); title('MIM'); xlabel('AAL region'); ylabel('AAL region');
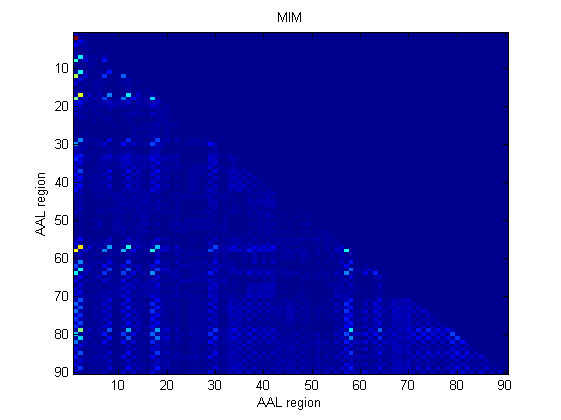
Which regions have the strongest connection?
[mmp, idxm] = max(mimaal(:));
[im, jm] = ind2sub(size(mimaal), idxm);
fprintf('Strongest AAL connection: %s <-> %s \n', cell2mat(tl(im(1))), cell2mat(tl(jm(1))));
Strongest AAL connection: Precentral_R <-> Precentral_L
6. MIM on sensor level
An additional idea to use the Multivariate Interaction Measure is to compute the interaction between two sets of electrodes, e.g. each hemisphere, and to visualize the corresponding spatial patterns:
% electrodes in left hemisphere + middle idx1=sa.locs_2D(:,2)<mean(sa.locs_2D(:,2)); % figure; % showfield(zeros(sum(idx1),1),sa.locs_2D(idx1,:)); % electrodes in right hemisphere idx2=~idx1; % figure; % showfield(zeros(sum(idx2),1),sa.locs_2D(idx2,:)); data1=data(:,idx1); data2=data(:,idx2); data12=[data1 data2]; % Calculating the cross-spectrum and coherency [csall]=data2cs_event(data12, segleng, segshift, epleng, maxfreqbin, para); [cs1]=data2cs_event(data1, segleng, segshift, epleng, maxfreqbin, para); % or alternatively: % cs12=csall(1:sum(idx1),1:sum(idx1),:); % proof: % unique(cs1-cs12) [cs2]=data2cs_event(data2, segleng, segshift, epleng, maxfreqbin, para); % or alternatively: % cs22=csall(sum(idx1)+1:end,sum(idx1)+1:end,:); % proof: % unique(cs2-cs22) % Here, 7 and 4 are quite arbitrary parameter to control for overfitting [mim,mic,a,b]=proc_mim(csall(:,:,idxg),sum(idx1),sum(idx2), 7, 4);
Now, the spatial filters a,b are transformed into interpretable patterns, see:
Haufe et. al, On the interpretation of weight vectors of linear models in multivariate neuroimaging, NeuroImage, Volume 87, 15 February 2014, Pages 96-110, ISSN 1053-8119, http://dx.doi.org/10.1016/j.neuroimage.2013.10.067.
This can be done as follows:
% please note that the sign of the patterns is arbitrary. % Therfore, abs() is used t1=(real(cs1(:,:,idxg)))*abs(a); t2=(real(cs2(:,:,idxg)))*abs(b);
Visualizing the spatial patterns reveal information about the underlying sources. Please note that the spatial patterns are theoretically not unique. They basically define a subspace of spatial patterns. To make them unique, additional assumptions are required, e.g. spatial non-overlap as implemented in:
L. Marzetti, C. D. Gratta, and G. Nolte. Understanding brain connectivity from EEG data by identifying systems composed of interacting sources. Neuroimage, 42(1):87–98, 2008
Here, however, the spatial patterns reveal valid information about the underlying sources:
figure; subplot(1,2,1) showfield(t1,sa.locs_2D(idx1,:)); subplot(1,2,2) showfield(t2,sa.locs_2D(idx2,:));
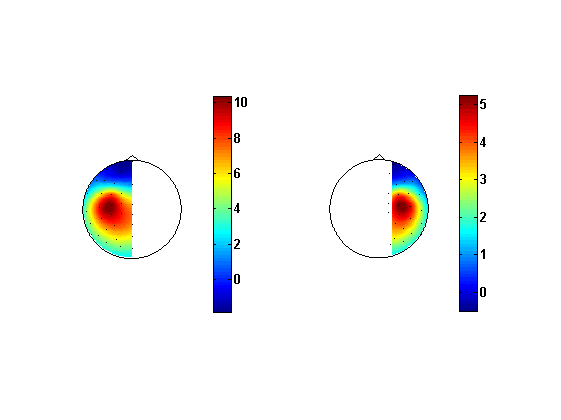
7. Wedge MUSIC
Given multiple sources (actually more than 2), e.g. from SC-MUSIC, it might not be clear which source is interacting with which source (in terms of the imaginary part of coherency). This problem can be approach with Wedge Music which is a hybrid source localization and connectivity approach.
A. Ewald, F. S. Avarvand, and G. Nolte., Wedge MUSIC: A novel approach to examine experimental differences of brain source connectivity patterns from EEG/MEG data. NeuroImage. 101:610–624, ISSN 1095-9572, 2014. http://dx.doi.org/10.1016/j.neuroimage.2014.07.011
Wedge MUSIC scan
In the following, the sources estimated with SC-MUSIC, are used as reference sources and a scan over all voxels is executed to determine the source which is interacting with the respective reference source.
wmscan=zeros(nv,ns); alpha1=zeros(nv,ndim, ns); for i=1:ns [wmscan(:,i), alpha1(:,:,i)]=wedgemusic_scan(cs(:,:,idxg),sa.V_coarse,vmax_all(:,i),ns); end
Plotting the results: In each figure, the source in the upper row is the SC-MUSIC source and the source in the lower row is the Wedge Music source
source_pars = struct('orientation', 'all', ... 'nsubplotrows', 2, ... 'colorbars', 1); climsSC=zeros(ns,2); climsWedge=zeros(ns,2); for i=1:ns fig=figure; % SC MUSIC Source (Seed) in upper row ... source_pars.subplotstart=0; res_sc=1./(1-(s_all(:,i))); source_pars.mricenter=true_dipoles(1,1:3); showmri_transp_v31(sa.mri,source_pars,[sa.grid_coarse,res_sc], true_dipoles); % is "interacting" with the % Wegde MUSIC Source in the lower row [~, idxm]=max(wmscan(:,i)); source_pars.mricenter=true_dipoles(1,1:3); source_pars.subplotstart=4; showmri_transp_v31(sa.mri,source_pars,[sa.grid_coarse,wmscan(:,i)], true_dipoles); end
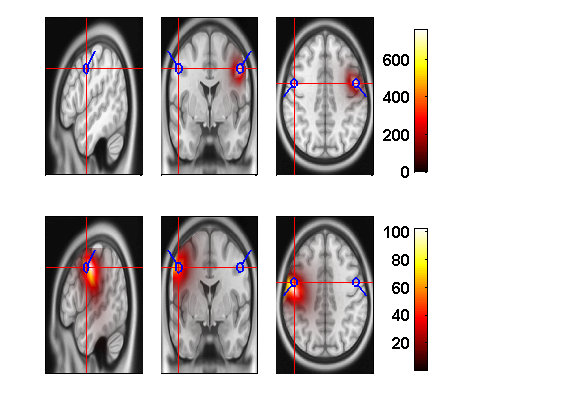
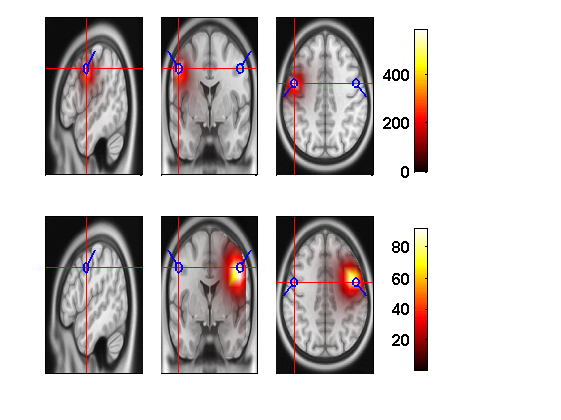
scalar Wedge MUSIC
A. Ewald, F. S. Avarvand, and G. Nolte., Wedge MUSIC: A novel approach to examine experimental differences of brain source connectivity patterns from EEG/MEG data. NeuroImage. 101:610–624, ISSN 1095-9572, 2014. http://dx.doi.org/10.1016/j.neuroimage.2014.07.011
% this is the scalar Wedge MUSIC result between the source topographies % of the sources obtained by SC MUSIC [wmsc_true, a1]=wedgemusic_scalar(cs(:,:,idxg),vmax_all(:,1),vmax_all(:,2),ns); % this is the scalar Wedge MUSIC result between two random vectors [wmsc_rand, a2]=wedgemusic_scalar(cs(:,:,idxg),randn(nch,1),randn(nch,1),ns); fprintf('\nScalar Wegde MUSIC between the two SC MUSIC sources: %2.4f. \n', wmsc_true); fprintf('Scalar Wegde MUSIC between one true SC MUSIC source and a random vector: %2.4f.\n', wmsc_rand);
Scalar Wegde MUSIC between the two SC MUSIC sources: 41.7705. Scalar Wegde MUSIC between one true SC MUSIC source and a random vector: 1.0017.
complete Wedge MUSIC
Here, Wedge MUSIC is performed for each pair of voxels WARNING: Takes long, approx. 15-20 Minutes
% A. Ewald, F. S. Avarvand, and G. Nolte., Wedge MUSIC: A novel approach % to examine experimental differences of brain source connectivity patterns % from EEG/MEG data. NeuroImage. 101:610–624, ISSN 1095-9572, 2014. % http://dx.doi.org/10.1016/j.neuroimage.2014.07.011
fprintf('Performing complete Wedge MUSIC. Might take a while...\n');
tic [wmcomp, al]=wedgemusic_complete(cs(:,:,idxg),sa.V_coarse,imax_all(1),ns); toc
% plot source_pars=[]; source_pars = struct('orientation', 'all', ... 'nsubplotrows', 2, ... 'colorbars', 1); [m, idxm]=max(wmcomp); source_pars.mricenter=ceil(sa.grid_coarse(idxm,:)); figure; showmri_transp_v31(sa.mri,source_pars,[sa.grid_coarse,wmcomp]);